Introduction
Generative AI is taking the digital world by storm today, and businesses are using it to conduct operations. It has become a pillar of innovation, from creating personalised customer experiences to automating complex workflows. However, to realize its full potential at scale, it requires a robust technological foundation.
So, which technologies must be in place to use large-scale generative AI for business? Let’s explore.
Table of Contents
1. Key Technologies for Large-Scale Generative AI
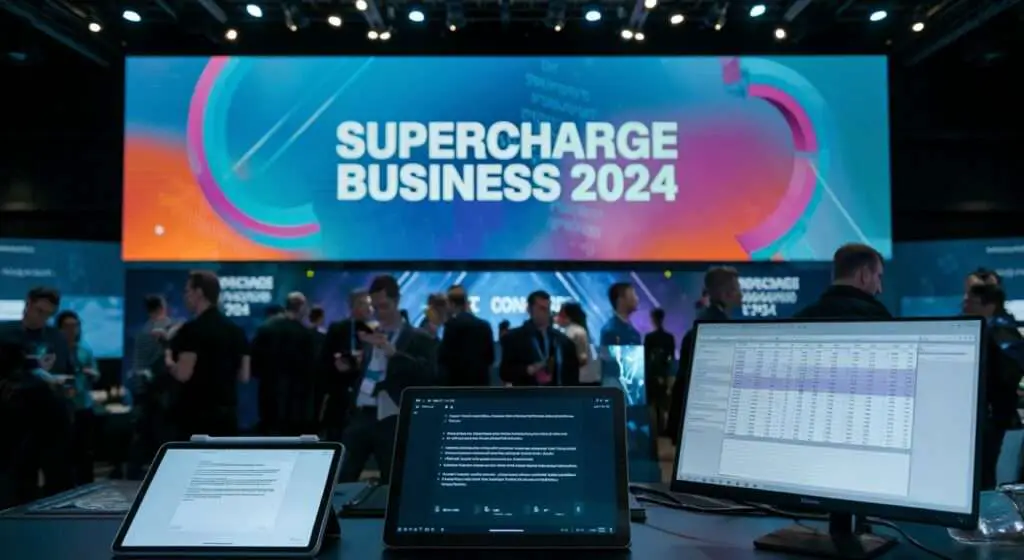
To make it work, generative AI needs powerful computing systems. For maximum results, businesses need to invest in high performance infrastructure. This helps create the perfect AI operations and efficiency.
Essential Components for AI Performance
- Powerful Hardware: For generative AI models to work smoothly, they need robust hardware like multi-core processors, GPUs, and AI-specific accelerators. These tools help speed up tasks such as training models and handling large datasets efficiently.
- Scalability: The computing power needed grows as the need for AI grows. With a scalable setup, businesses can continue to scale their AI operations without slowing down, and perform smoothly and grow.
- Reliability: Trustworthy systems make sure AI applications run the same way all the time, without unexpected hiccups. Confidence can be built with reliable infrastructure which keeps users’ attention directed towards innovation.
Without these systems, it’s challenging to address the question, “Which technologies must be in place to use large-scale generative AI for business?”
2. Data Storage and Processing
While generative AI is built on data, it is that data that powers its functionality. Failing systems for storing and processing this data is a critical problem. Keeping these systems efficient helps keep AI performance and accuracy.
Importance of Data Management in AI
- Ensure Reliability: Large datasets in AI require scalable storage solutions to make your systems both reliable and efficient. Maintaining the smooth operation of AI models requires good data management.
- Enhance Performance: Keeping AI models accurate is all about organizing and accessing your data efficiently. However, simple data management practices can really boost the performance of your AI system.
- Maintain Integrity: By keeping the integrity of your data intact you maintain the trust and accuracy of AI outputs. Building and maintaining trust in AI technologies requires consistent and clear data management practices.
This process underscores which technologies must be in place to use large-scale generative AI for business, as data is the fuel that powers AI.
3. Machine Learning Frameworks
Creating generative AI models require machine learning frameworks. And they provide the tools and libraries for development. An efficient and reliable AI solution is guaranteed by these resources.
Benefits of Popular AI Frameworks
- User-Friendly Platforms:
There are easy to use interfaces from TensorFlow, PyTorch and MXNet. These make it possible to develop and train AI models for everyone.
- Versatile Applications:
These frameworks provide support for a long list of AI solutions. They can be easily customized to fit unique needs and goals for businesses.
- Trustworthy and Reliable:
These tools are backed by experts in the tech industry to make sure they are robustly performing. They can be trusted by developers to develop high quality AI models.
Understanding which technologies must be in place to use large-scale generative AI for business starts with having the right development tools.
4. Deep Learning Architectures
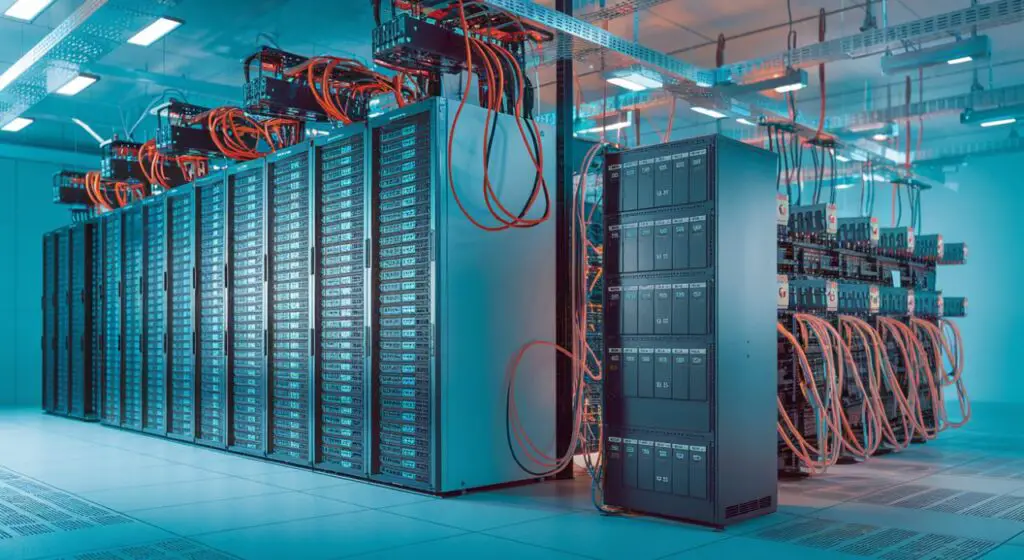
Generative AI is driven by innovation from deep learning architectures. These advanced models let machines make original content. Find out how AI creativity is changing the face of industries today.
Common Models
- Convolutional Neural Networks (CNNs): Image recognition is a strong use case of CNNs that serve as the backbone for generative applications. Because they are flexible they are perfect for these tasks to create new visuals.
- Recurrent Neural Networks (RNNs): Because RNNs are good at understanding sequences, they are ideal for text synthesis. They are able to predict and even generate text, and are now a staple of generative tech.
- Generative Adversarial Networks (GANs): In the process of training two models to outsmart each other, GANs produce some stunning, realistic content. As a result, the outputs are very creative, ideal for media and design.
When considering which technologies must be in place to use large-scale generative AI for business, deep learning architectures are non-negotiable.
5. Natural Language Processing (NLP)
The ability to understand and generate language is important to AI applications. These skills are what allows machines to interact with people naturally. Learning about them increases the effectiveness of AI at real world tasks.
Understanding AI with NLP
- AI chatbots and virtual assistants are basically language processing in their blood, allowing them to understand and speak human language without having to worry about it. This tech simplifies and enhances the interactions of users.
- NLP allows businesses to go deep into customer feedback and learn how to improve your products and services. It’s like having a smart assistant to help improve user satisfaction and engagement.
- Why NLP isn’t just about text: building trustworthy AI systems users can trust. How AI learns by analysing language makes it credible to tackle real world tasks.
Having a clear grasp of which technologies must be in place to use large-scale generative AI for business ensures businesses fully leverage language-based AI tools.
6. Cloud Computing
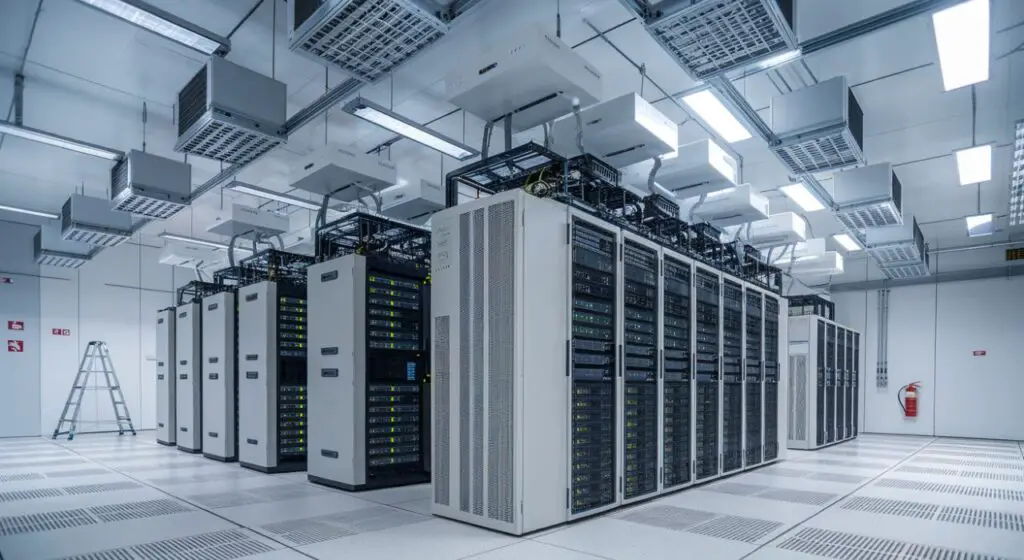
Deployment of AI is highly required by cloud computing for its scalability and flexibility. It helps businesses to deal with large quantities of data and complicated algorithms. Cloud solutions come with trust, meaning they are reliable and robust AI integration.
Benefits of Cloud Platforms for AI Applications
- Flexible Infrastructure: Thanks to cloud platforms like AWS, Azure, and Google Cloud, it is easy to set up and businesses can adjust their resource based on their AI needs without hassle.
- Cost-Effective Solutions: Using cloud services allows businesses to avoid the expense of buying expensive on site equipment which is a smart way to save money and be more efficient.
- Easy Scalability: Cloud platforms come in handy for companies to easily expand their operations on AI without any hassle of growth and adaptation as demands increase, while still maintaining top notch performance.
Cloud solutions address the question of which technologies must be in place to use large-scale generative AI for business, offering flexibility and cost savings.
7. Data Security and Privacy Measures
A key focus is on AI security with expertise and trust. Building confidence in technology, it is important to do safe AI practices. Keep up to date with the measures for security required in protecting AI systems properly.
Ensuring Data Privacy and Risk Management
- Building trust and reliability in your AI solutions while remaining compliant with regulations like GDPR, is of utmost importance in protecting your users’ sensitive data. Maintaining that trust means robust security is a non negotiable.
- To succeed with AI deployment, we need to prevent data breaches and to use the content generated by AI responsibly. It’s a way to reassure users their data is safe and their experience is important.
- As AI solutions become more transparent about how data is used, and what measures are in place to protect it, trustworthiness grows. Security practices get more user confidence with clear communication about them.
Answering which technologies must be in place to use large-scale generative AI for business means prioritizing data security and privacy at every step.
8. Integration with Existing Systems
Integrating with existing systems, generative AI is revolutionizing business by making things work seamlessly. It uses its capabilities to improve efficiency and innovating. Learn how this technology can elevate your business operations.
Interoperability
- Seamless workflows and better efficiency can only be achieved when you integrate AI with older systems. This makes sure everything works together all the time without a hitch.
- Interoperability helps you increase productivity by ensuring that your software is on the same page. Running your business like a well oiled machine is what it helps you do.
- There is no need to throw out your legacy systems; instead, you just need to add AI. This approach saves time and money and modernizes your processes.
Knowing which technologies must be in place to use large-scale generative AI for business helps streamline integration efforts.
9. Talent Acquisition and Training
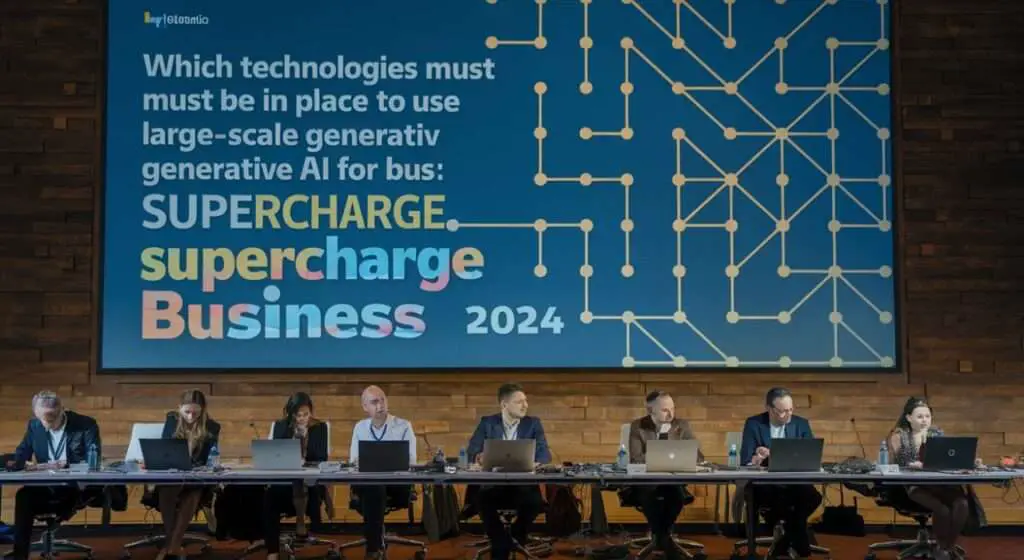
People make technology advance. People with their expertise and creativity always try to break the barrier and innovate. This is all about trust in human capability.
Building a Skilled AI Workforce
- In order to develop and sustain advanced AI systems, finding the right AI experts is vitally important. Any successful AI project is powered by these talented individuals.
- Help your team to stay ahead in the world of AI by encouraging continuous learning. Uptskilling staff will keep your business competitive and innovative.
- You can trust your team’s expertise to provide reliable AI solutions. A confident workforce leads to better outcomes with a knowledgeable workforce.
A skilled workforce understands which technologies must be in place to use large-scale generative AI for business and ensures smooth implementation.
10. Ethical Guidelines and Governance
Responsible Technology Use depends on ethics in the AI deployment. It makes sure that AI systems are safe, fair and transparent. The ethics of AI helps build trust and expertise in modern tech rollout.
Responsible Use and Governance Frameworks
- Without ethical guidelines, AI can be biased and manipulative, which means that the technology can’t be for everyone. If we define clear rules, we can direct AI development in a responsible direction.
- With a strong governance framework, AI is made accountable, with transparency around how a decision is made. It builds trust among users and stakeholders that helps more reliable use of AI.
- Helping better outcomes come from expert insights and authoritative voices in AI management. When experts are at the front of the line, users and developers alike feel comfortable in the technology.
Addressing which technologies must be in place to use large-scale generative AI for business includes adhering to ethical and governance standards.
Conclusion
Implementing large-scale generative AI in business is no small feat. It requires careful planning, resources, and expertise. Businesses need to know which technologies must be in place to use large-scale generative AI for business.
It includes bringing together the most complex AI technologies, training personnel, and equipping AI strategies with business objectives. Organizations that are investing in the right technologies are able to use AI’s transformative power to grow, innovate and increase customer satisfaction.
Processes can be automated and valuable insights can be uncovered from data and personalized customer experiences created, which will help businesses to stand out in the market.
FAQs
What is generative AI and how does it benefit businesses?
Generative AI creates content such as text, images, or even code: enhancing creativity and efficiency.
Why is high-performance computing crucial for generative AI?
It gives the computational power needed to process complex models and datasets.
What role does data security play in generative AI?
Protecting sensitive data will ensure compliance and establish trust with customers.
How can businesses ensure ethical AI deployment?
Through clear guidelines and governance frameworks.
What is generative AI and how does it benefit businesses?
The primary obstacles are high costs, ethical concerns, and technological complexity.
People also ask
What are the key benefits of using generative AI in business operations?
It generates AI that increases efficiency through task automation, improves decision-making with data insights, and fosters innovation with creative solutions.
How can generative AI improve customer experience in enterprises?
It personalizes the interactions by providing instant support through chatbots and creating tailored content that raises customer satisfaction and loyalty.
What are the main challenges in implementing generative AI for businesses?
Its major challenges include ensuring data privacy, managing ethical considerations, and integrating AI into the existing systems effectively.
How do high-performance computing and machine learning frameworks work together in generative AI?
They collaborate to process large data sets quickly, enabling complex AI models to learn and generate high-quality outputs efficiently.
What are the best practices for scaling generative AI in large enterprises?
Start with pilot projects, ensure robust data infrastructure, and continuously monitor and refine AI models for optimal performance.