What is machine learning and how has it contributed to advancements in AI? Machine learning (ML) is the area of artificial intelligence (AI) that focuses on enabling computers to learn from data and improve their ability to perform tasks over time without explicit programming.
This has been the driving force behind the major advancements made in AI, changing the way we use and interact with technology across many industries.
Table of Contents
1. Definition of Machine Learning
Machine learning is the way computers learn and improve over time by studying data, without needing constant human instructions. It’s one of the key parts of AI, driving changes in healthcare, finance, and even how we use smartphones.
This technology helps systems make smarter decisions, faster than ever before.
Understanding the Concept
- Core Idea: Machine learning is training algorithms with statistical models to give a computer the ability to learn from and make predictions on data.
- Types of Learning:
- Supervised Learning: Supervised Learning: It uses labeled datasets for training models for classification or prediction tasks.
- Unsupervised Learning: It finds patterns in data without predefined labels.
- Reinforcement Learning: Agents learn to make decisions through trial and error. They receive feedback in the form of rewards or penalties.
2. Key Advancements Driven by Machine Learning
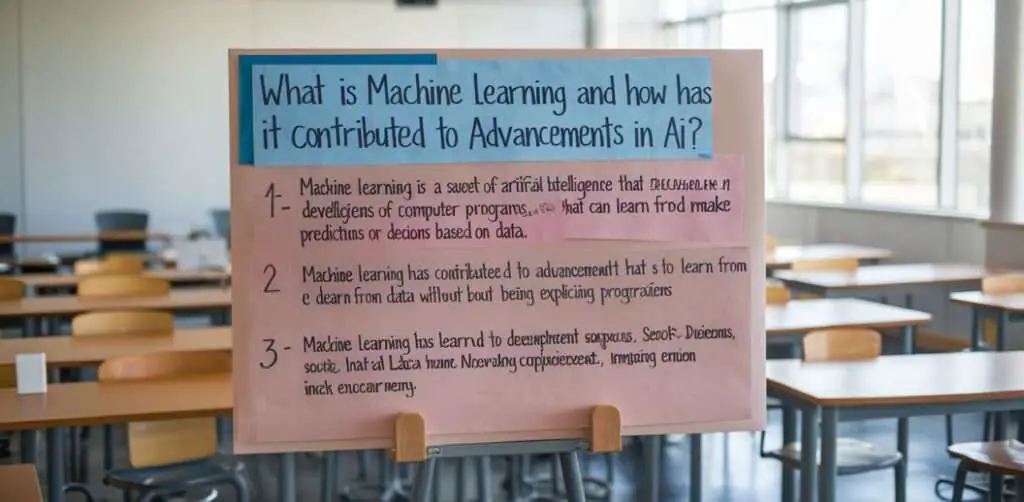
What is machine learning and how has it contributed to advancements in AI? Machine learning is a type of artificial intelligence that teaches computers how to learn from data and evolve without constant human input, powering technologies such as virtual assistants, fraud detection, and medical diagnoses.
Because it uses smart algorithms that solve problems faster and in greater accuracy than traditional solutions, machine learning is seen as a key driver for the advancements we are observing today in AI.
Transformative Applications
- Healthcare: ML algorithms scan medical images for diagnostic purposes, predict patient outcomes, and fine-tune treatment plans, all enhancing patient care.
- Finance:In banking, ML recognizes fraudulent transactions through pattern recognition in large volumes of data, thereby enhancing risk management and operational efficiency.
- Transportation: Self-driving cars rely on ML to make instantaneous decisions based on sensory inputs, leading to a great impact on road safety and navigation.
3. Enhancements in Natural Language Processing (NLP)
Machine learning boosts AI, teaching computers to learn and improve from data without human instructions. It makes life smarter and more efficient by powering innovations in healthcare, finance, and transportation.
Improving Human-Machine Interaction
- Conversational AI: Advanced NLP models that are powered by ML help machines understand and generate human language, thus enabling a way of interaction through chatbots and virtual assistants.
- Translation Services: Deep learning techniques enhance the accuracy of language translation tools, breaking down barriers in communication globally.
4. Image Recognition and Computer Vision
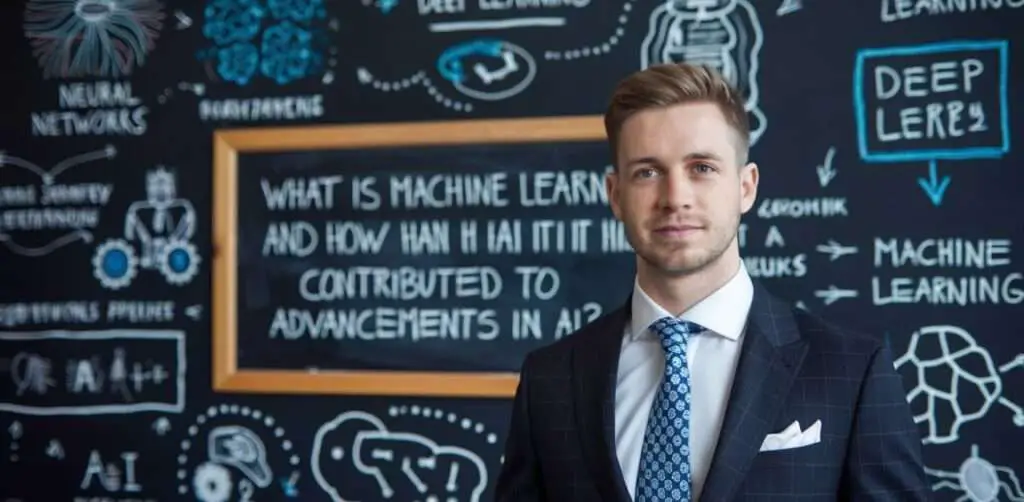
Machine learning is one of the most potent aspects of artificial intelligence: computers can learn from data and improve over time without requiring constant programming. It drives innovations such as smarter virtual assistants, fraud prevention, and life-saving medical tools.
Visual Data Processing
- Deep Learning Models: CNNs are very effective in the processing of visual information that results in tremendous breakthroughs in facial recognition, object detection, diagnostics in the domain of medical imaging diagnostics.
- Applications:These are used in most industries of security (surveillance), health care diagnostic imaging.
5. Automation of Routine Tasks
This branch of artificial intelligence gives computers the ability to learn and improve without being manually reprogrammed. Machine learning has made possible the most mundane solutions: virtual assistants, self-driving cars, fraud detection systems.
Increasing Efficiency
- Business Process Automation: It automates such repetitive tasks across industries freeing human resources for strategic tasks.
- Recommendation Systems: E-commerce applications use machine learning algorithms to personalize user experiences and even suggest products to customers on the basis of past behavior.
6. Continuous Improvement Through Data
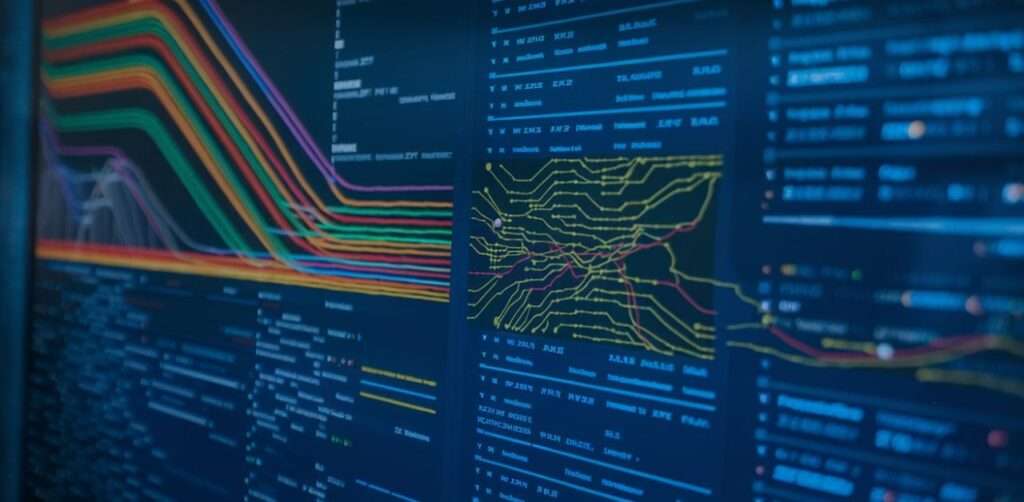
Machine learning is a subset of artificial intelligence that enables computers to learn from data, improve by themselves, and work automatically without human intervention. Some of the popular applications include virtual assistants, fraud detection, and self-driving cars.
Learning from Experience
- Feedback Loops: ML systems are constantly learning from new data inputs in order to improve the algorithm for better accuracy and performance over time.
- Big Data Utilization: The ability to analyze large datasets allows organizations to extract insights that were previously unattainable.
7. Challenges and Ethical Considerations
Machine learning is the most advanced technology that enables computers to learn from data and get better with time without being constantly programmed. It has revolutionized industries such as healthcare, finance, and transportation by solving problems faster and more accurately.
Addressing Concerns
- Bias in Algorithms: Ensuring fairness is critical to ML systems, as bias results in discrimination.
- Data Privacy Issues: Collection and utilization of personal data can compromise user privacy and safety.
Conclusion
What is machine learning and how has it contributed to advancements in AI? Machine learning is a key part of artificial intelligence that has completely transformed many industries. By enabling machines to learn from data and make smart decisions, it has brought significant progress to areas like healthcare, finance, transportation, and more.
This technology enhances efficiency and creates better user experiences, making it an essential driver of modern advancements.
As we continue to see technology grow, it’s important to tackle challenges like bias and privacy. Addressing these issues will be crucial for the responsible development of machine learning applications, ensuring they benefit everyone fairly and ethically.
Frequently Asked Questions
How do CNNs and RNNs differ in their applications?
CNNs (Convolutional Neural Networks) are ideal for image and video analysis, while RNNs (Recurrent Neural Networks) excel at processing sequential data like text and speech. They serve different tasks based on data structure.
What are some real-world applications of reinforcement learning?
Reinforcement learning is used in robotics for automation, gaming for AI opponents, and finance for stock trading strategies. It helps systems learn through trial and error.
How has NLP improved translation services?
NLP (Natural Language Processing) has made translation services more accurate by understanding context, idioms, and grammar. This ensures smoother and more natural translations.
What industries benefit most from machine learning?
Industries like healthcare, finance, e-commerce, and manufacturing benefit the most, using machine learning for better predictions, automation, and customer personalization.
How does machine learning enhance predictive maintenance?
Machine learning predicts equipment failures by analyzing data patterns, reducing downtime, and saving costs. It ensures timely maintenance and improved efficiency.
People also ask
1. How does machine learning contribute to AI?
Machine learning powers AI by enabling systems to learn from data and make decisions without being explicitly programmed. It helps AI adapt, improve, and perform tasks like image recognition and language translation more effectively.
2. How do AI, ML, and deep learning contribute to advancements in technology?
AI, ML, and deep learning drive innovation by automating tasks, improving accuracy, and enabling smarter systems. They push advancements in healthcare, finance, transportation, and more through predictive analytics and automation.
3. What are some examples of AI and machine learning in everyday life?
AI and machine learning are everywhere—voice assistants like Alexa, recommendation systems on Netflix, spam filters in emails, and personalized ads are all examples. These technologies make daily life easier and more personalized.
4. What is the difference between machine learning and AI?
AI is the broader concept of machines mimicking human intelligence, while machine learning is a subset of AI that focuses on systems learning and improving from data. ML is how AI gets smarter over time.
5. What is the main goal of machine learning?
The main goal of machine learning is to create systems that learn from data and improve their performance over time without human intervention. This helps solve complex problems and automate decision-making.